Smart Tech News
by Mary Vincent
12 December 2022
New Book: No Miracles Needed - How Today's Technology Can Save Our Climate and Clean Our Air by Mark Z. Jacobson
18 October 2022
Scaling AI Summit
07 March 2022
New Book: Let It Shine: The 6,000-Year Story of Solar Energy
Smart Tech News would like to recommend a NEW Book for our Readers:
Let It Shine: The 6,000-Year Story of Solar Energy by John Perlin paperback edition features a new preface by the author and a foreword by Stanford Professor Mark Z. Jacobson, PhD. The Book:
- Features a new preface by the author, detailing the explosive growth of solar since the publication of the cloth edition of Let It Shine in 2013
- The price of solar modules has dropped by 90 percent since 2010, and installation has grown exponentially
- Solar panels are now the cheapest energy source in history
- Features a new foreword by Stanford professor Mark Z. Jacobson, a pioneer in developing road maps to transition states and countries to 100 percent clean, renewable energy
What People are saying:
“The definitive history of solar power.”
— The Financial Times
“John Perlin is the historian of solar energy.
— Daniel Yergin, Pulitzer Prize–winning author of The Prize and The Quest
More about the book:
Even as concern over climate change and dropping prices fuel a massive boom in solar technology, many still think of solar as a twentieth-century wonder. Few realize that the first photovoltaic array appeared on a New York City rooftop in 1884, or that brilliant engineers in France were using solar power in the 1860s to run steam engines, or that in 1901 an ostrich farmer in Southern California used a single solar engine to irrigate three hundred acres of citrus trees. Fewer still know that Leonardo da Vinci planned to make his fortune by building half-mile-long mirrors to heat water, or that the Bronze Age Chinese used hand-size solar- concentrating mirrors to light fires the way we use matches and lighters today.
Let It Shine is a fully revised and expanded edition of A Golden Thread, John Perlin’s classic history of solar technology, detailing the past forty-plus years of technological developments driving today’s solar renaissance. This unique and compelling compendium of humankind’s solar ideas tells the fascinating story of how our predecessors throughout time, again and again, have applied the sun to better their lives — and how we can too.
You can buy it at your local bookstore and here is one store from San Francisco.
Enjoy!!
30 August 2020
Winners of the 2020 IoT World Awards Announced
16 winners of the 2020 IoT World Awards were selected from 600-plus nominees.
Winners of the second-annual IoT World Awards were announced on Wednesday, August 12, 2020, at the Internet of Things World conference. The awards series celebrates innovative individuals, teams, organizations and partnerships that advance IoT technologies, deployments and ecosystems.
This year, there were more than 600 nominations for the awards. Entrants spanned the Internet of Things ecosystem, from industrial IoT technology to edge computing and consumer offerings, as well as deployments in several industry sectors. More than 80 companies and individuals were selected as finalists before 16 winners were crowned.
A panel of judges from Omdia, Informa Tech and the industry chose the winners of the core awards based on the entries’ innovation, market traction and other factors. The panel evaluated nominations in January 2020, and the IoT World Awards shortlist was published in February 2020. Two leadership awards were also selected based on votes from almost 5,000 industry professionals.
IoT World Today and IoT World Series also introduced the COVID-19 Innovation Award, recognizing companies for their work in combating the novel coronavirus. This award was judged separately by a smaller panel in July 2020.
The complete list of winners of this year’s competition follows:
Technologies
- Industrial IoT Solution: Zebra End-to-End Supply Chain Visibility
- Edge Computing Solution: FogHorn Lightning Edge AI Platform
- IoT Connectivity Solution: STMicroelectronics STM32WLE
- IoT Platform: Software AG Cumulocity IoT
- IoT Security Solution: Darktrace Enterprise Immune System
- Consumer IoT Solution: AWS IoT for Connected Home
Deployments
- Manufacturing IoT Deployment: AGCO Component Manufacturing Using a digital execution system from Proceedix
- Energy IoT Deployment: Saudi Aramco’s camera-based Auto Well Space Out
- Healthcare IoT Deployment: En-route online point-of-care testing service for the London Ambulance Service
- Public Sector IoT Deployment: Libelium flexible sensor platform and Terralytix Edge Buoy
- Consumer IoT Deployment: Kinetic Secure by F-Secure, Windstream and Actiontec
Ecosystem Development
- IoT Partnership of the Year: iBASIS Global Access for Things
- Startup of the Year: Latent AI: Latent AI Efficient Inference Platform
COVID-19 IoT Innovation Award
- Igor Nexos Intelligent Disinfection System
Enterprise Leader of the Year
- Deanna Kovar, vice president, production and precision agriculture production systems at John Deere
IoT Solutions Leader of the Year
- Aleksander Poniewierski, global IoT leader at EY
10 March 2020
08 March 2020
Make AI Explanations Everyone Can Understand
Why asking an AI to explain itself can make things worse
Creating neural networks that are more transparent can lead us to over-trust them. The solution might be to change how they explain themselves.
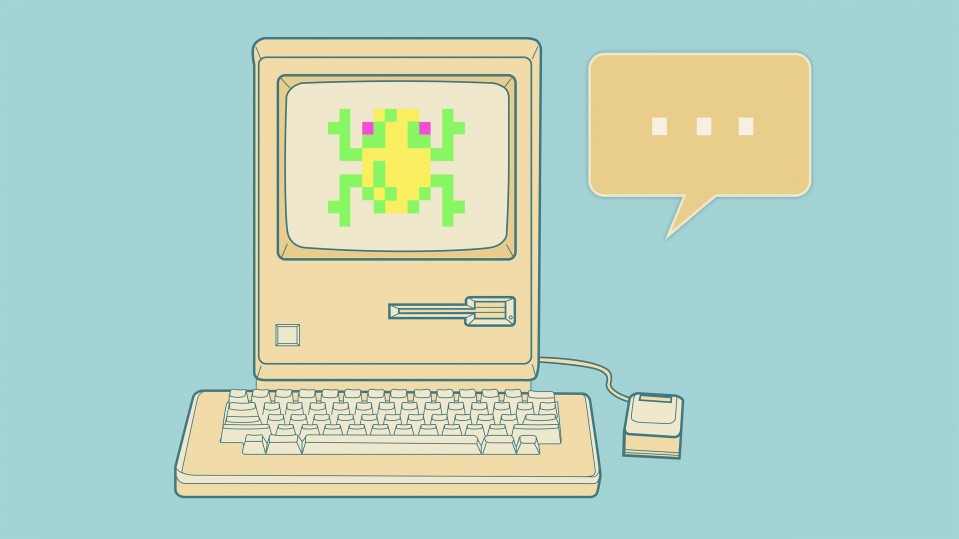
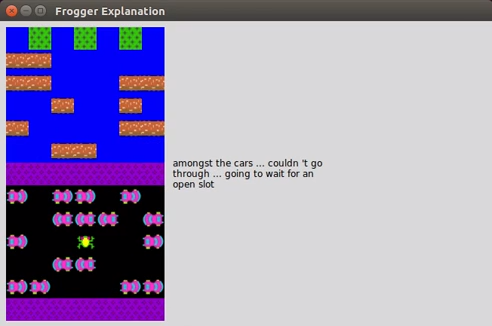